December 2, 2019
Expert: How to make deep learning as energy efficient as the brain
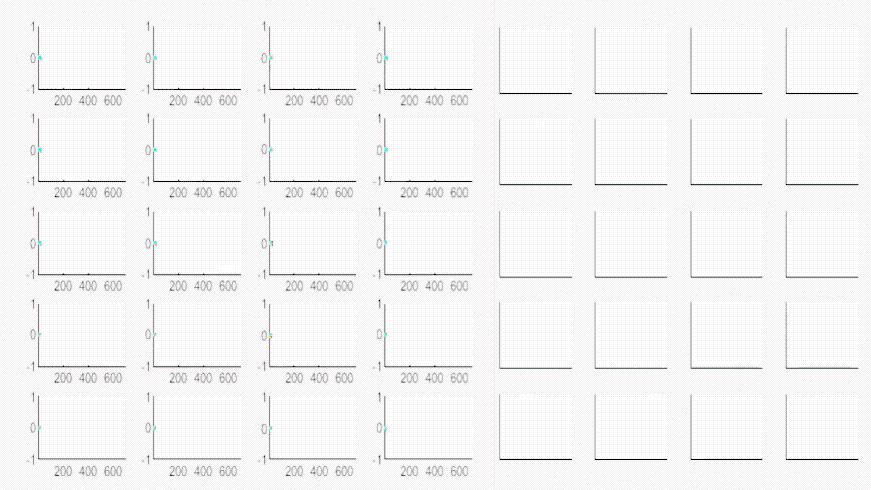
WHAT: Computers are gradually thinking like humans thanks to the development of artificial intelligence networks capable of learning on their own, called “deep learning.” These networks can already recognize images and play chess, for example.
But in comparison to the human brain, deep learning can require up to 1,000 times more energy to perform the same functions. This means that if smart glasses used deep learning to recognize objects, the battery would last only 25 minutes, studies have shown.
In a perspective paper published in Nature, Purdue University researchers recommend that deep-learning networks mimic electrical signals in the brain, called “spikes,” to be more energy efficient.
EXPERT: Spikes are mostly how the brain computes, says Kaushik Roy, Purdue’s Edward G. Tiedemann Jr. Distinguished Professor of Electrical and Computer Engineering and director of the Center for Brain-Inspired Computing (C-BRIC)
When a spike from on neuron reaches another neuron and hits a certain threshold, the neuron fires, performing a computation. Information is stored in a connecting structure, called a synapse, between neurons. Roy believes that the brain only computes and updates this information when spikes come, allowing the brain to save energy.
Roy’s lab has been creating algorithms and devices that mimic these spiking neurons and synapses. They also develop ways to train computers using spikes, such as to write numbers as instructed by a voice or to play a game, demonstrating that spike-based learning is possible.
QUOTE: “Deep learning has made enormous progress, and in most cases it performs better than humans. But at what cost? Turns out that cost is a huge energy gap.
“We know we can’t have the hardware of the brain, but if we could take cues from the brain to design hardware using silicon, it’s possible that deep learning could perform even better and on far less energy.”
Writer: Kayla Wiles, 765-494-2432, wiles5@purdue.edu
Source: Kaushik Roy, 765-494-2361, kaushik@ecn.purdue.edu
Note to Journalists: For a copy of the Nature paper, please contact Kayla Wiles, Purdue News Service, at wiles5@purdue.edu.