September 2, 2020
Purdue Engineering receives Department of Energy funding to speed up adoption of 3D-printed microreactors
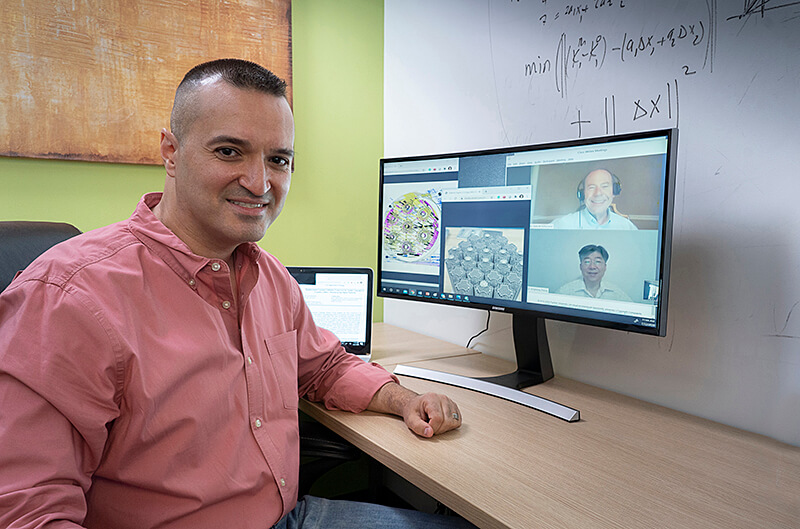
Hany Abdel-Khalik meets virtually with John W. Sutherland (top on screen), and Xinghang Zhang.
WEST LAFAYETTE, Ind. – The U.S. Department of Energy has awarded an $800,000 grant to Purdue University's College of Engineering for a project to accelerate the introduction of the 3D-printed microreactor – a new type of nuclear reactor with the flexibility and versatility needed for many current energy applications.
The Nuclear Energy University Program funding will enable Purdue to be a key contributor to the Transformational Challenge Reactor Demonstration Program, in which the Department of Energy’s Oak Ridge National Laboratory is working to build the first 3D-printed microreactor by 2023. The microreactor also will be the first advanced reactor to operate in the U.S. in more than 40 years. To support this mission, Purdue will develop and demonstrate a novel artificial intelligence method to ensure the quality of the microreactor’s components.
The Purdue project is intended to drive the use of additive manufacturing (also known as 3D printing), computation materials modeling, and AI concepts in creating nuclear reactor components. Goals of all these technologies are to significantly reduce manufacturing costs and development time, and to realistically estimate safety risks while offering reliability and convenient access to nuclear power.
“Microreactors introduce a transformational trend to the nuclear industry – a trend that enables more streamlined construction and deployment processes to address the nation’s energy challenges that cannot be overcome solely with large-scale nuclear reactors,” said Hany Abdel-Khalik, technical lead for the project and associate professor of nuclear engineering.
“Purdue will fill a technological gap in the nuclear industry, reflecting a broader trend of applying AI strategies to support additive manufacturing (AM). AM enables designs to be adjusted during manufacturing, greatly decreasing production cost and time. Our work is aimed at driving widespread adoption of additively manufactured reactor components by using an AI-powered software system to ensure safety and reliability.”
Kurt Terrani, director of the TCR program at ORNL, said, “Synchronized application of additive manufacturing and artificial intelligence techniques are key to providing the most data-rich and cost-effective nuclear component qualification process. This is one of the key goals of DOE-NE’s TCR program: using modern technology to deliver a new and better way to deploy nuclear energy.
“The program is engaging the industry, the regulator and, in this case, universities in order to ensure an optimal approach is developed and adopted in widespread fashion. The technical strength of the Purdue team will shore up our ability to deliver on these goals.”
“Purdue Engineering has ideal strengths to support the mission of the Department of Energy on the development of additively manufactured microreactors,” said project leader John W. Sutherland, the Fehsenfeld Family Head of Environmental and Ecological Engineering. “We bring together deep expertise and collaborative capabilities, encompassing manufacturing, nuclear engineering, materials engineering, environmental engineering, AI and data analytics, nuclear reactors modeling and simulation, and systems engineering. In this project, we’ll be blending fundamental science and engineering in a very complex and dynamic environment.”
The project team also includes Steven Shade, the Ball Brothers Director of Advanced Manufacturing Initiatives, who is affiliated with Environmental and Ecological Engineering, and Xinghang Zhang, co-principal investigator and professor of materials engineering, with a background in AM and other areas of manufacturing.
Zhang said Purdue’s solution will apply reinforcement learning, a kind of AI that uses advanced machine learning strategies to fine-tune the selection of the optimum AM process parameters – such as printing speed and melting temperature in this case – to train the AI models and guide decision making.
Source: Hany Abdel-Khalik, abdelkhalik@purdue.edu