Engineers develop faster, more accurate AI algorithm for improving nuclear reactor performance
Purdue University research reactor serves as test bed for optimizing performance of small modular reactors
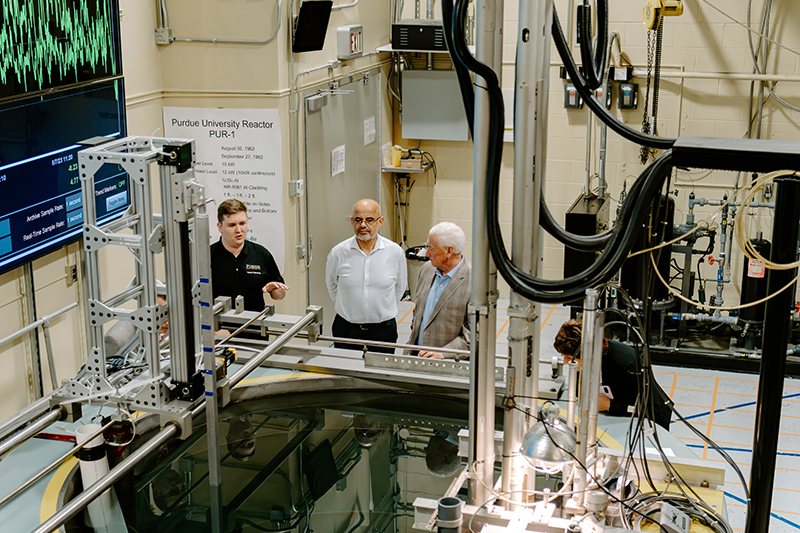
Rep. Greg Pence, a member of the U.S. House Energy and Commerce Committee (second from right), toured Purdue University Reactor Number One, Indiana’s first and only nuclear reactor, alongside Purdue engineering faculty and research leaders in August 2023. (Purdue University photo/Kelsey Lefever)
WEST LAFAYETTE, Ind. — To expand the availability of electricity generated from nuclear power, several countries have started developing designs for small modular reactors (SMRs), which could take less time and money to construct compared to existing reactors.
Toward this effort, a study conducted at Purdue University has made progress in enabling artificial intelligence to improve monitoring and control of SMRs, possibly offering a way to further cut costs of their operation and maintenance so that they can be more economically viable.
The study, published in Nature’s Scientific Reports, showed how a machine learning algorithm could rapidly learn about the physics behind a measurement of how steadily a reactor is producing power, and predict changes in this indicator over time with 99% accuracy.
ADDITIONAL INFORMATION
- Purdue University chosen by Indiana Office of Energy Development for small modular nuclear reactor study
- DOE Undersecretary Richmond visits Purdue nuclear and fluid power facilities
- Greg Pence joins Purdue leadership, faculty to discuss innovation in energy research
- Feasibility study: Advanced nuclear technology is a potential option to achieve zero carbon emissions for Purdue University
The researchers believe that an algorithm like this one, which doesn’t require as much training as other AI methods that have been proposed for predicting a reactor’s performance, might help engineers achieve efficient reactor monitoring and control. It could also allow future operators to monitor and improve a reactor’s performance more effectively over its whole lifespan.
Researchers from Purdue and the U.S. Department of Energy’s (DOE) Argonne National Laboratory conducted this study using measurements from the Purdue University Reactor Number One (PUR-1), the first and only reactor in the U.S. licensed with a fully digital instrumentation and control system.
Most existing reactors have analog systems, limiting how much AI can benefit their operation. SMRs, on the other hand, will have digital gauges and sensors, opening a door for AI to collect real-time data and inform their design and performance more comprehensively.
PUR-1’s digital instrumentation and controls make it an ideal test bed for developing and testing AI algorithms.
“We can harmoniously couple the subatomic world with AI systems that can dig into these numbers and extract all kinds of nuggets of information and knowledge about maintaining and improving the machine,” said Konstantinos Prantikos, the first author of this paper and a graduate research assistant in Purdue’s School of Nuclear Engineering.
Only used for educational and research purposes, PUR-1 also is the site of the first “digital twin” nuclear reactor control system on a university campus, which Purdue and Argonne researchers used to conduct this study. The digital twin is a clone of PUR-1 that allows researchers to collect real-time data and realistically simulate the reactor on a computer and experiment without affecting the reactor’s operation. The data is available in real time through a virtual program, which can then be used to view and analyze the data.
“With a digital twin, it’s possible to develop the capability to monitor a reactor remotely. In the future, SMRs could use digital twins to have algorithms running in the background that can predict what’s going to happen in the next minute, in the next hour, and then provide information to the operator to make adjustments,” said Stylianos Chatzidakis, a Purdue assistant professor of nuclear engineering and associate director of PUR-1, whose research group created the reactor’s digital twin.
The Purdue-Argonne team tested the machine learning algorithm’s ability to monitor and predict fluctuations in the number of neutrons released from the reactor core. These neutrons kick-start fission, which is the chemical reaction that allows a reactor to produce power. The reaction relies on neutrons to continuously split uranium-235 atoms, releasing a high volume of energy. Nuclear power plants use this energy to generate electricity.
The algorithm can predict neutron flux for PUR-1 with an error rate below 1% on average, which is sufficient accuracy for monitoring a reactor, the researchers report.
This accuracy is due to how comprehensively the algorithm learned about the reactor’s neutron flux levels. The researchers designed the algorithm to learn from a physics model trained on neutron flux level measurements provided via the digital twin. The algorithm then made predictions on how these levels could change.
The team found that this transfer of learning between the physics model and machine learning algorithm not only improves accuracy, but also happens in just a few seconds, significantly cutting down the training time needed to develop an algorithm that can monitor and improve the performance of a reactor.
This work builds on previous collaborations between Argonne and Purdue. The two entered into an agreement last year that allows certain researchers to share affiliations to both institutions. Under this joint appointment master agreement, designated researchers will have access to facilities and expertise at both the national laboratory and Purdue. Alexander Heifetz, a principal nuclear engineer at Argonne who collaborated with Purdue on this study, is a visiting scholar in Purdue’s School of Nuclear Engineering through this agreement.
This research is funded by DOE’s Advanced Research Projects Agency-Energy and a donation from Goldman Sachs Gives to the AI Systems Lab at Purdue.
About Purdue University
Purdue University is a public research institution demonstrating excellence at scale. Ranked among top 10 public universities and with two colleges in the top four in the United States, Purdue discovers and disseminates knowledge with a quality and at a scale second to none. More than 105,000 students study at Purdue across modalities and locations, including nearly 50,000 in person on the West Lafayette campus. Committed to affordability and accessibility, Purdue’s main campus has frozen tuition 13 years in a row. See how Purdue never stops in the persistent pursuit of the next giant leap — including its first comprehensive urban campus in Indianapolis, the Mitchell E. Daniels, Jr. School of Business, Purdue Computes and the One Health initiative — at https://www.purdue.edu/president/strategic-initiatives.
Sources have limited availability. Please contact Kayla Albert for interviews.
Paper
Physics-informed neural network with transfer learning (TL-PINN) based on domain similarity measure for prediction of nuclear reactor transients
Scientific Reports
https://doi.org/10.1038/s41598-023-43325-1
The abstract and author list are available online.
Writer/Media contact: Kayla Albert, 765-494-2432, wiles5@purdue.edu