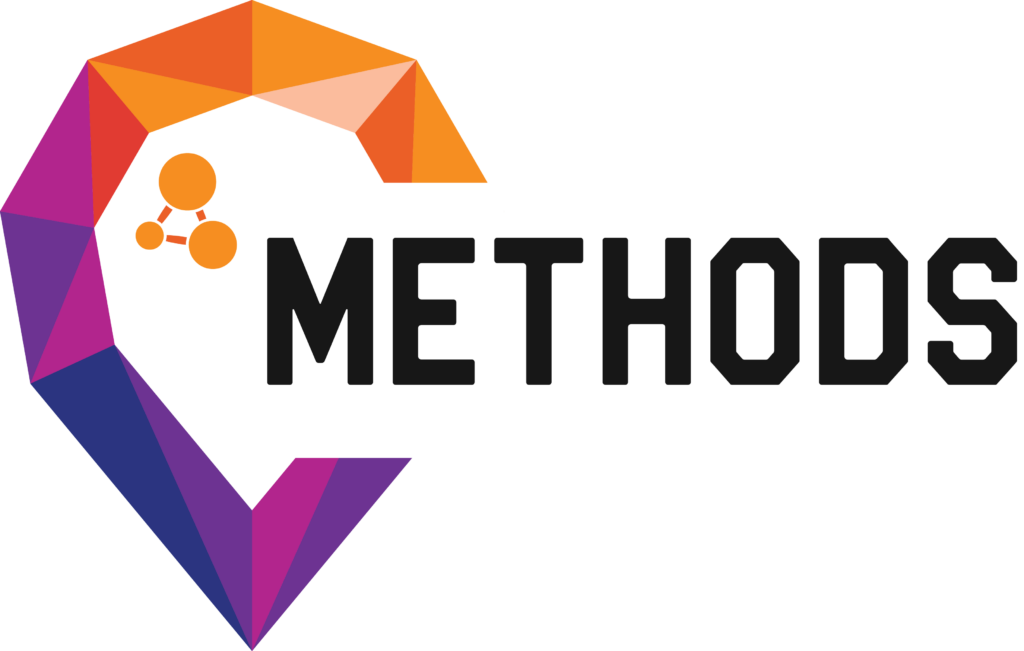
Machine Learning Enabled Two-Phase Flow Metrologies, Models, and Optimized Designs
An Office of Naval Research (ONR)
Multidisciplinary University Research Initiative (MURI)
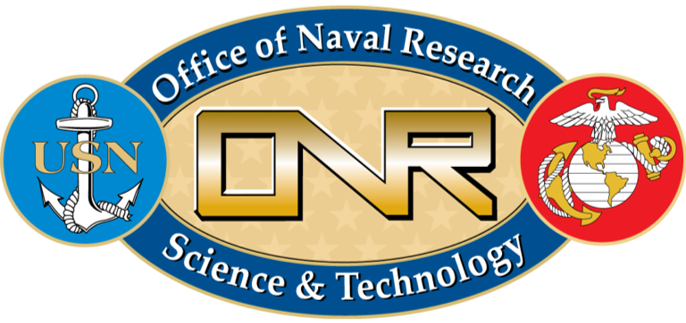
Program Research Thrusts
Thrust 1
Novel optical metrology and advanced image processing will yield experimental datasets with unprecedented spatial resolution visualizing the dynamics of flow boiling. Essential for model validation and training, these data will include phase and temperature distributions at wall surfaces, thin slices of phase and liquid film thickness distributions, and velocity and temperature fields. The techniques will transform the ability of optical metrologies to probe interfaces, temperatures, and velocities in two-phase flows owing to novel structured illumination schemes, molecular tagging diagnostics, and 3D data augmentation techniques.
Thrust 2
Deep learning models will detect features in first-of-their-kind empirical data to allow training of PhI ML-based two-phase models. Object detection models will extract detailed statistical information on two-phase flow features to improve understanding of phase-change behavior and its impact on performance. Gray-box models will discover enhanced, thoroughly validated formulations to eliminate empiricism from conservation equations. Training of interfacial transport relations using a physics informed neural network framework, coupled with numerical simulations, will deliver the ability to perform two-phase computational fluid dynamics.
Thrust 3
Deep neural operator surrogates will predict two-phase flow at full complexity with high efficiency and uncertainty quantification. To ensure model accessibility, integration within a natural language design framework will offer a virtual assistant to optimize components and system designs. Reduced order models will enable robust two-phase flow forecasting, equipping the design assistant with capabilities to control thermal systems.
