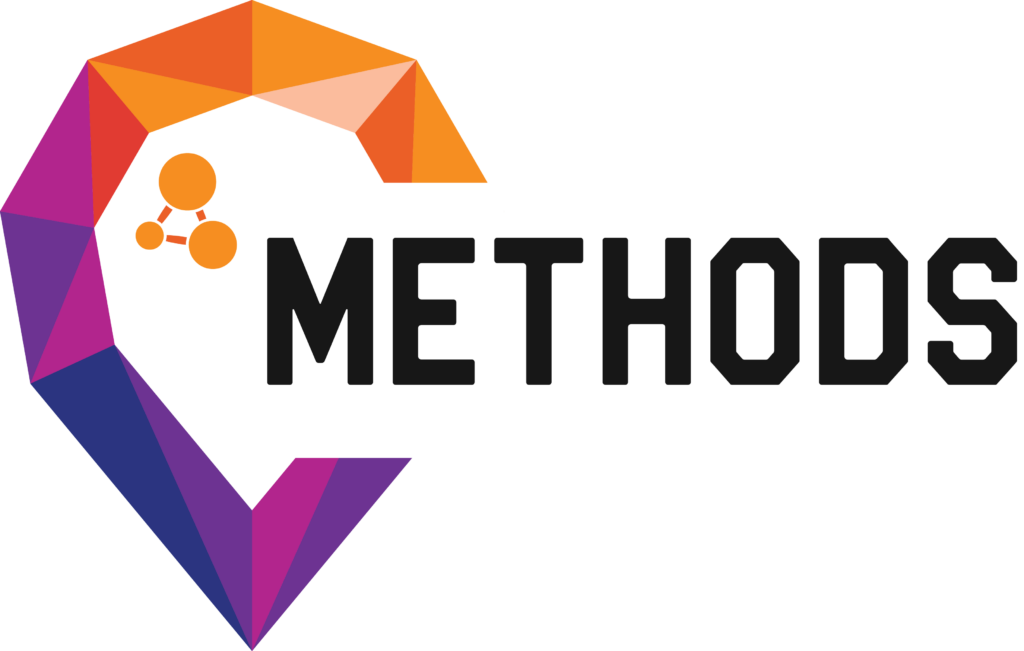
Machine Learning Enabled Two-Phase Flow Metrologies, Models, and Optimized Designs
An Office of Naval Research (ONR)
Multidisciplinary University Research Initiative (MURI)
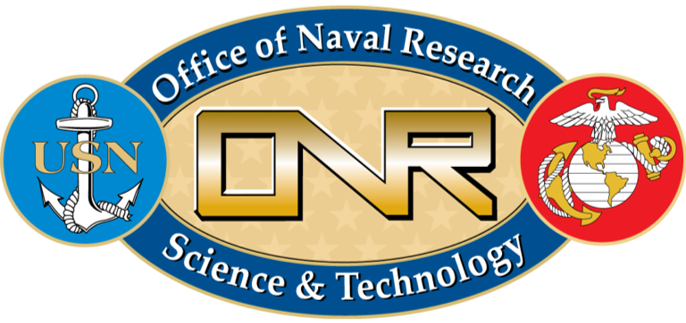
Advanced metrology, modeling, and design for two-phase flows and heat transfer

Thermal management systems that leverage liquid-to-vapor phase change have the potential for substantial size, weight, and power (SWaP) advantages compared to existing systems. However, the design and optimization of two-phase systems is hindered by the inability to model and predict flow and heat transfer with high certainty and generality. Established mechanistic models remain inherently empirical and have good prediction accuracy only for specific flow regimes, geometries, or fluid types. Furthermore, these models are developed and validated using a few global parameters, despite awareness that performance is governed by local spatiotemporal phenomena. Even when high-fidelity data are available, heuristic interpretation has limited physical insights and feedback into the model development process.
To address these gaps, a multidisciplinary convergence of liquid-vapor phase change transport modeling domain knowledge, advanced fluid physics metrology, and emerging physics-informed (PhI) machine learning (ML) and computer vision techniques can provide a long-sought unified modeling “language” to predict two-phase flow and heat transfer. The goal of METHODS (Machine learning Enabled Two-pHase flow metrologies, models, and Optimized DesignS) is to leverage PhI ML approaches to enhance fundamental understanding and develop an end-to-end framework to predict phase change during two-phase flows.
The envisioned generalized framework for characterizing, modeling, and predicting two-phase flows will provide a toolset that can transform the ability to engineer two-phase systems to their full potential. With access to generalized predictions across all operating conditions and transients, SWaP benefits of two-phase thermal management systems can be safely realized in applications of interest without requiring extensive hardware design build-test cycles.
